Subscribe to the blog
Process modelling for chromatography – how is it used?
April 27, 2020 / The Bio-Works Team
Process modelling of chromatographic behavior, in an industrial setting, can be a useful tool in dealing with the complexities of purification process development, optimization and scale-up. It can be used to identify critical process parameters and support process design.
While it is not feasible to explore all process parameters for each step, in a traditional process development setting based on lab experiments and statistical models such as DoE, mechanistic process models can supplement available experimental data and aid in the design of specific experiments. Experimentation can be reduced, saving time and cost during process development
All in all, it can contribute to a better understanding and performance of chromatography processes.
Biopharmaceutical drug purification continues to be expensive, time-consuming and risky
There have now been several decades of effort invested in improving the efficiency and performance of the processes involved in bringing a biopharmaceutical product to market. But biopharmaceuticals are complex substances and their purification presents significant extra challenges beyond those for purifying small molecule drugs.
The development of chromatography of a biopharmaceutical involves in-depth exploration of an extremely large parameter space (typically 50–100 operating parameters and material attributes impact performance of a chromatography process) to develop optimal, safe, and economic processes.
For example, for the commonly used ion-exchange chromatography, buffer pH and ionic strength, gradient, stationary phase, column ionic capacity, resin properties, protein properties, and buffer conditions can affect the product yield as well as the final product quality.
Nowadays, it is mostly experimentation and interpretation of results using experience and judgement that drive development of downstream processes. Amongst other challenges, the amount of material available for testing is often limited, and timelines are constricted due to pressure to reduce time-to-market. US Food and Drugs Administration (FDA) regulations require the basic separation scheme to be decided on early in the drug development process, prior to starting clinical trials.
The FDA encourages use of quality by design (QbD) principles during process development and operation
Quality by Design (QbD) is a science- and risk-based approach, outlined in the International Conference on Harmonization (ICH) Q8(R2), Q9, and Q10, Q11 and soon Q12. These guidelines provide the foundation for implementing QbD during specific stages of product development.
QbD begins with identification of product requirements that will meet patient needs followed by determination of the quality target product profile (QTPP) and the Critical Quality Attributes (CQAs) required to meet those needs. The substance, the manufacturing process, and quality control strategy are then designed to ensure the desired product quality, for example purity, is consistently met.
Within the QbD framework, a thorough understanding of process inputs and their impact on performance is therefore key, in particular the relationship between the process parameters and the products’ CQA, and the association between the CQA's and a product's clinical properties.
The QbD approach is expected to increase assurance of product quality, and in turn, the FDA will grant manufacturers greater flexibility to operate with a lower regulatory burden, enabling continuous process improvement, as well as greater robustness.
Process modeling is important for future biopharmaceutical chromatography process development within QbD.
Process modelling is a useful tool to study the impact of various process parameters on process performance and product CQAs. Some process parameters are difficult or even impossible to alter experimentally but it is trivial to assess them by modelling. In the typical industrial setting, both time and material are limited, so it is valuable to perform simulations quickly. The lessons learnt can contribute to better understanding as well as reduced processing risks.
The use of modeling to support the design of effective and efficient manufacturing chromatography processes that will ensure a predefined product quality is entirely consistent with the basic tenet of Quality by Design.
Modeling can be used to assess impact of input variables, to make process robustness studies more efficient, aid process monitoring, and to facilitate implementation of process analytical technology (PAT).
The real value of process modelling in the kind of complex operations that chromatography represents, is to contribute to a systematic approach in process development, monitoring, and control.
Different types of modeling are suitable for various tasks in process development
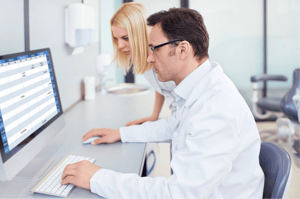
A basic requirement for a good theoretical model is that it is predictive and explains at least most of the data variability, but it must also be feasible to implement in an industrial environment. Models allowing for an extrapolation beyond the calibration range are most powerful, as they can be used for process scale-up, transfer from batch to continuous operations, and to predict the impact of e.g. changed feedstocks coming from upstream processing.
Commonly used modelling approaches are of two broad types:
- Empirical modeling - uses experimental data e.g. based on design of experiments (DOE).
DOE-based approaches are the most common type of modelling in industry and can be quite effective at providing process insights and determining a scale-dependent design space. On the other hand, they lack accuracy and robustness as they do not incorporate the underlying mechanisms. As they are unable to extrapolate beyond the calibration range, DOEs need to be designed for a specific purpose (e.g. optimization of the load phase of a chromatography step) and frequently they cannot be used for any other task.
In practice, DOEs is easy to implement in industry with the help of commercial software such as MODDE™ or Jmp!™.
- Mechanistic modeling - built on fundamental physico-chemical principles of the system.
Based on the existing physico-chemical understanding of chromatography, mechanistic models can be calibrated with a much smaller set of experimental data, typically 5-15. The calibrated models provide a mechanistic process understanding because the model parameters have a physical meaning and translate the effect of process parameters and material on the products’ CQAs.
In mechanistic modeling, there is a strict separation of model calibration and model application. Once the model is calibrated, it can be applied to all tasks in process development, characterization, or even root-cause investigation in manufacturing.
The challenge in implementing mechanistic modeling in industry is the complexity of the underlying theory. To minimize the effort for model calibration, simulation software such as ChromX™ are used in companies that specialize in mechanistic chromatography modeling and extract model parameters directly from chromatograms.
Final remarks
Resin structures and chemistry will continue to evolve, and many advancements have been made in the past decade that have made it more feasible to use mechanistic modeling to achieve efficient manufacturing. Presumably the industry will see a rise in modeling during the next decade as the biotech industry incorporates the principles of QbD and PAT and moves towards continuous processing.
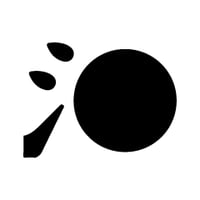